Safety Challenges For Generative AI Agents In Autonomous Machines
Generative AI will be increasingly integrated into autonomous machines delivering an agentic element.
Hence transitioning from virtual applications like chatbots to systems with physical capabilities and physical embodiment.
Introduction
This evolution heightens existing safety issues such as hallucinations and harmful outputs while introducing new challenges.
These include catastrophic forgetting, real-time processing demands, resource constraints, lack of formal safety guarantees, and limited real-world grounding in all instances.
These factors make safety-critical considerations significantly more urgent for AI systems with physical autonomy.
But, how epic is this diagram? At the extreme left we have the genesis, Generative AI, with four model types. Of late, Multi-Modal Language Models and Foundation Models have fuelled the advent of Agency (AI Agents, Agentic X).
This is due to the fact these models have vision, and hence interaction with environment elements are possible. Section 4 shows the different applications with Section 5 listing the safety challenges.
AI agents provide unparalleled capabilities, they also have unique safety concerns.
Generative Systems
Generative AI systems are becoming valuable in autonomous systems, where their context-aware reasoning and semantic and symbolic understanding create breakthroughs in navigation, perception, and task planning and execution.
Considering semantic and symbolic understanding, I still feel not enough attention has been given to the symbolic reasoning capabilities of models. It is the symbolic understanding of LLMs that allows the model to create a mind/mental map it navigate tasks with.
Multi-Modal Language Models vs Foundation Models
According to the study, the key difference between multi-modal language models and foundation models lies in their focus and scale:
Multi-Modal Language Models integrate multiple data types (e.g., text, images, audio) and align them to process inputs like image-text pairs for tasks such as captioning and visual question answering. They emphasize cross-modal understanding and interaction.
Foundation models, in contrast, are extremely large-scale models trained on vast datasets, often across various modalities, to generalise across tasks.
Their focus is on advanced contextual understanding, reasoning, and adaptability, making them foundational for diverse applications, including robotics and AI systems.
I guess this is debatable and in some instances a model can be referred to in both terms. It feels like in future the general term to use will be Language Model.
Virtual & Physical AI Agents
Virtual Agency is something we have come to know very well in terms of chatbots and conversational UIs where our conversation systems lived within a digital environment and medium.
What is interesting is how the study describes this digital container as an action space is limited to digital tasks such as generating text, images, or music.
Digital AI Agents have control over virtual elements, but their actions do not directly influence the physical world.
The impact of these digital AI Agents remain confined to the digital realm.
Although significant, the risks posed by virtual agency are generally confined to non-physical, digital and data harms.
In contrast, physical agency has a larger and more complex action space.
Generative models in physical domains exert tangible influence, such as operating robotic arms, navigating vehicles, or preparing agents for real-world deployment through simulation.
Errors in these systems carry severe consequences, including physical damage, injury, or system failures.
Transitioning from virtual to physical environments magnifies the need for stringent safety measures due to the direct impact on the real world.
The study emphasises the necessity for specialised safety standards for generative AI with physical agency, exceeding those required in virtual-only applications.
Challenges
Generative models face significant challenges, particularly in safety-critical contexts.
Hallucinations, where models produce false or misleading outputs, pose risks when decisions rely on factual accuracy.
Catastrophic forgetting, where models lose previously learned knowledge during updates, can degrade performance in dynamic environments.
The absence of formal guarantees leaves no mathematical assurances for stability, safety, or reliability, making the systems unpredictable under certain conditions.
Models also lack real-world grounding, struggling to align with physical realities or practical constraints.
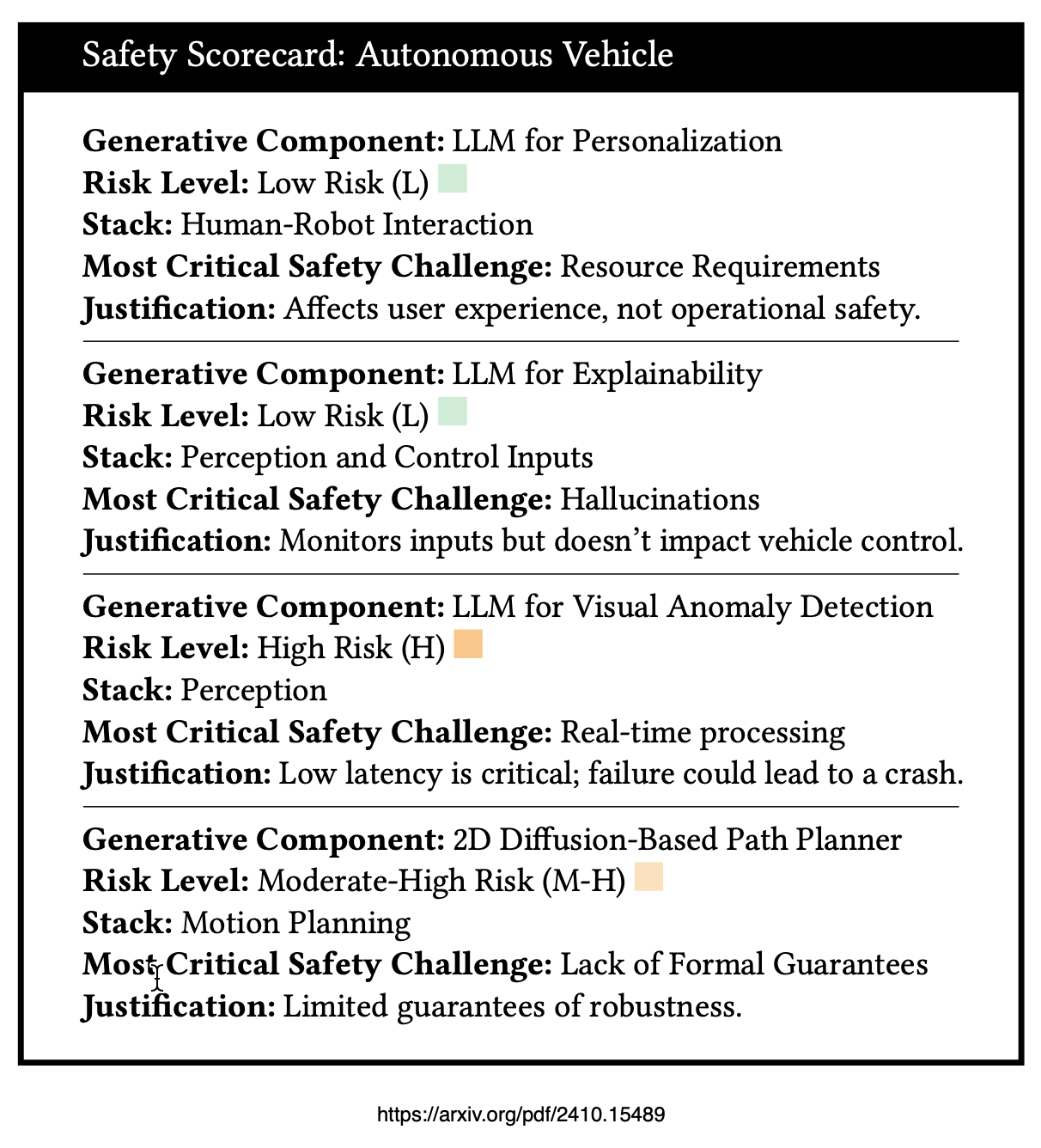
Resource-intensive requirements, including compute and energy, limit scalability and accessibility for diverse applications.
In physical domains, these challenges become critical as errors can lead to safety failures, physical harm, or operational risks.
To mitigate these threats, rigorous testing, safety frameworks, and robust training protocols are essential.
Without addressing these challenges, the integration of generative models into sensitive environments remains fraught with danger.
Chief Evangelist @ Kore.ai | I’m passionate about exploring the intersection of AI and language. From Language Models, AI Agents to Agentic Applications, Development Frameworks & Data-Centric Productivity Tools, I share insights and ideas on how these technologies are shaping the future.